What are the precisionFDA Truth Challenge V2 and CMRG, and how do they help us deliver better genomes?
Using whole-genome sequencing (WGS) technology and software developed at Illumina, nearly all the 6 billion nucleotides of a human genome can be analyzed with high accuracy and efficiency. These advances are driving a genomics revolution in health care, from investigations into the deep foundations of how our cells operate to precision medicine therapies targeting specific mutations.
The PrecisionFDA Truth Challenge V21 was designed to evaluate the state of the art of small variant calling, producing a benchmarking set covering 92% of the entire genome for three samples (HG002, HG003, and HG004). Illumina DRAGEN’s mapper and variant caller had the highest accuracy for Illumina reads in the Difficult-to-Map Regions and All Benchmark Regions categories, with 38% and 28% fewer call errors than the second-best contestants, respectively.
While the benchmarking callset released through the precisionFDA challenge focused on assessing accuracy in challenging regions such as difficult-to-map areas, segmental duplications, and the major histocompatibility complex (MHC) region, it is important to note that other areas of the human genome can be equally or even more challenging. Some variants and genes are resistant to standard methods of analysis and require a deeper look. These have become our focus as we strive to make whole-genome sequencing ever better. We’ve covered some of our work in previous blog posts, including methods to evaluate short tandem repeat expansions that cause conditions like Huntington disease2 and resolving the elusive GBA1 gene involved in risk for Parkinson disease and Gaucher disease.3
To empower benchmarking in the challenging regions of the human genome that matter most to human health, the United States National Institute of Standards and Technology (NIST) and collaborators at top institutions performing WGS published a list of “challenging medically relevant genes” (CMRG),4 an intersection between the two concepts of “medically relevant” and “technically challenging,” intended to be used as a consistent point of comparison across methodologies. In this way, CMRG both expands on the technical concept of difficult-to-map and narrows the focus to regions harboring variants associated with genetic diseases.
The medically relevant list includes genes curated by ClinGen, recommended by the American College of Medical Genetics for returning secondary findings, the Clinical Pharmacogenetics Implementation Consortium’s pharmacogenomics genes, and core genes for carrier screening recommended by the American College of Obstetricians and Gynecologists. These are genes that span a wide range of medical applications and can have a profound impact on human health throughout the lifespan.
“Challenging regions” have been defined by the Genome in a Bottle Consortium as regions where false negative and/or false positive results are observed when performing standard analyses on well-characterized cell line samples.
The precisionFDA Truth Challenge V2 and CMRG provide Illumina with datasets to measure accuracy from Illumina WGS data and provide an opportunity to demonstrate accuracy in regions that were once considered inaccessible. They highlight the benefit of coupling Illumina’s proven high-accuracy sequencing with DRAGEN’s innovative informatics to provide highly accurate variant calls across the genome.
This blog presents an evaluation of DRAGEN's germline small variant calling accuracy over multiple versions. Since 2020, DRAGEN methods have eliminated 70% and 60% of variant calling errors against the precisionFDA Truth Challenge V2 and CMRG, respectively. These results position DRAGEN as a highly compelling solution for germline variant calling and its associated applications.
Putting WGS and DRAGEN to the test
Accuracy on NIST v4.2.1 All Benchmark Regions
Figure 1 reports the accuracy of successive DRAGEN versions on all seven NIST samples5 for the v4.2.1 All Benchmark Regions, for SNP and indel combined. DRAGEN accuracy has significantly improved over time, with an average 71% error reduction over the past 2.5 years. The introduction of the multigenome (graph) reference and machine learning (ML) in versions 3.7.5 and 3.9.3, respectively, led to steep error reductions. But continuous improvements overall lead to ~70% error reduction in DRAGEN v4.2 compared to v3.4.5, and this improvement is consistent across all 7 NIST samples, regardless of ethnicity.
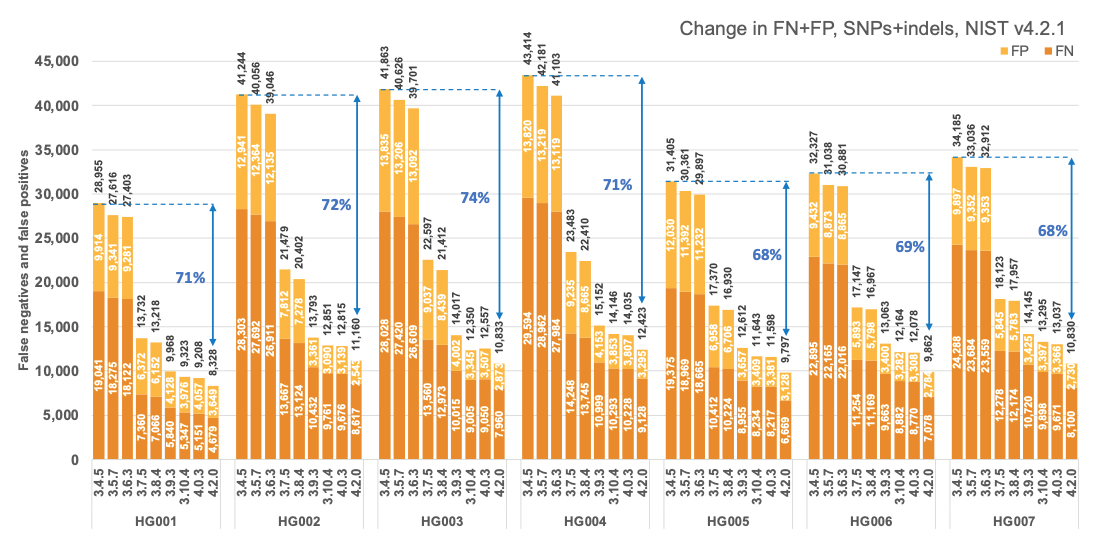
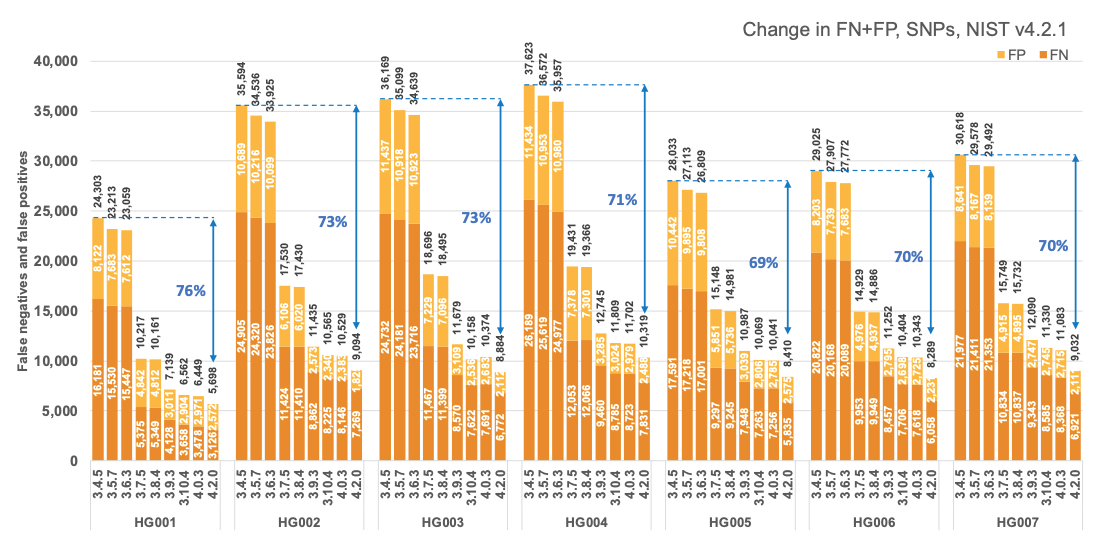
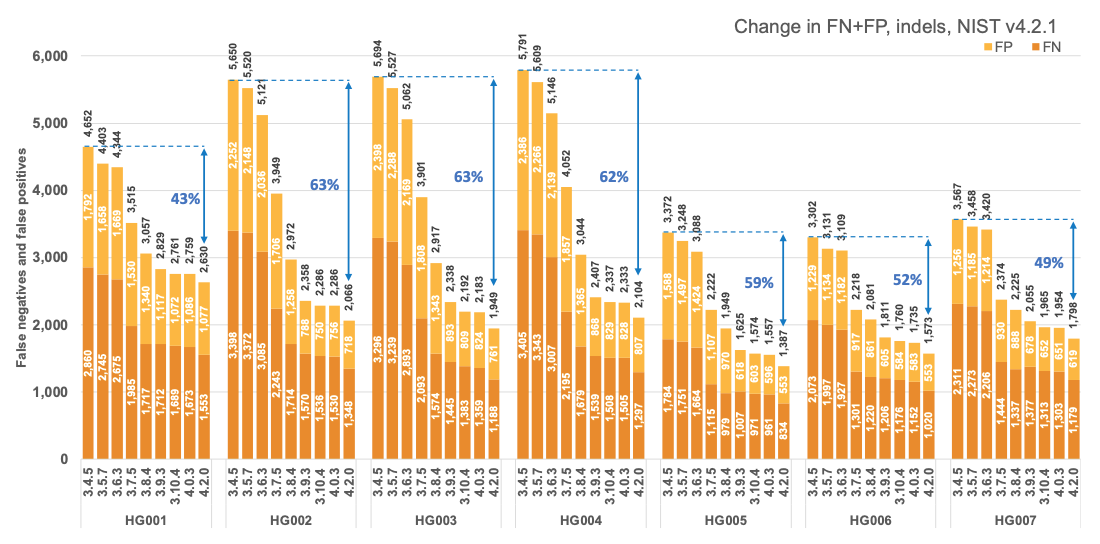
Accuracy comparison with selected pipelines from the precisionFDA V2 challenge
Figure 4 presents the accuracy of DRAGEN v4.2 on the precisionFDA V2 samples1 for the v4.2.1 All Benchmark Regions. This accuracy is compared to the accuracy of BWA-GATK6,7 and BWA-DeepVariant6,8 VCFs, which were obtained from the challenge results9 with submission designations “UYMUW” and “S7K7S,” respectively. In terms of error reduction, DRAGEN outperforms BWA-GATK by an average of 83% on combined SNPs and indels, with SNPs showing an average reduction of 84% and indels showing an average reduction of 79%. When compared to BWA-DeepVariant, DRAGEN shows an average error reduction of 60% on combined SNPs and indels, with SNPs showing an average reduction of 62% and indels showing an average reduction of 46%. These findings are consistent with published reports.10
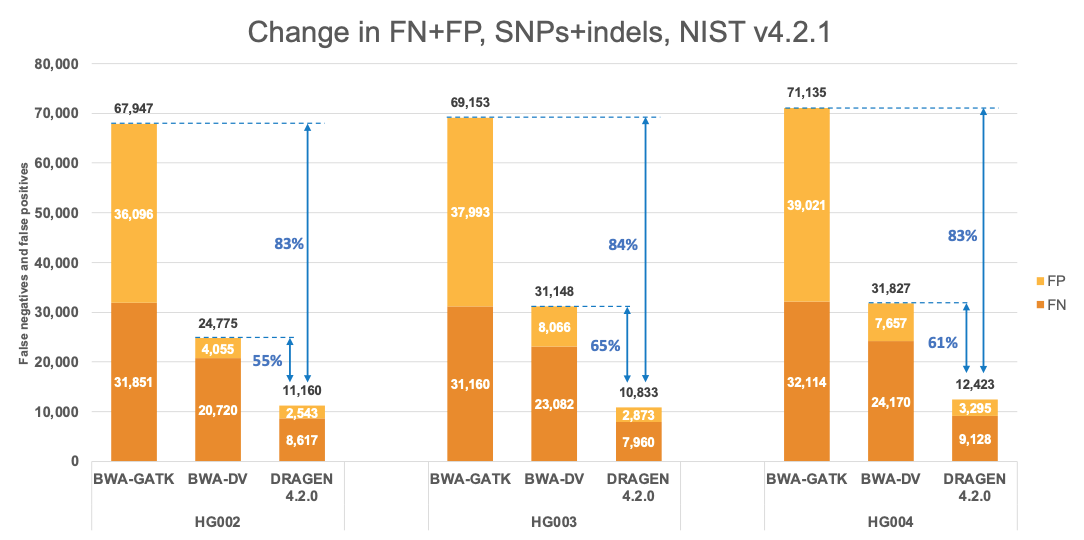
Accuracy comparison with selected pipelines from the Human Pangenome Reference Consortium evaluation
The Human Pangenome Reference Consortium (HPRC) recently released 47 high-quality assemblies of diverse human genomes selected to represent global genetic diversity.11 These 47 high-quality assemblies constitute the first draft of the human pangenome reference, which aims to reduce the reference bias introduced by single-genome references.
In Figure 5 and Table 1 we compare the accuracy of DRAGEN 4.2 to the accuracy obtained aligning on the HPRC reference pangenome with Giraffe12 and variant calling with DeepVariant and the BWA-DV pipeline on the HG001-HG007 samples for the v4.2.1 All Benchmark Regions. Both Giraffe-DV and BWA-DV VCFs were downloaded from Amazon S3.13 The samples used to generate the Giraffe-DV and BWA-DV VCFs were downsampled to 30x coverage; therefore, for a fair comparison, we downloaded the BWA BAM files and realigned the samples with both DRAGEN 3.7.5 and DRAGEN 4.2.0. When compared to BWA-DV, DRAGEN shows an average error reduction of 59% on combined SNPs and indels, with an average reduction of 61% on SNPs and 52% on indels, confirming the trend observed in the comparison showed on the precisionFDA V2 samples. When compared to Giraffe-DV, DRAGEN reports an average error reduction of 38% on combined SNPs and indels, with an average of 38% on SNPs and 41% on indels.
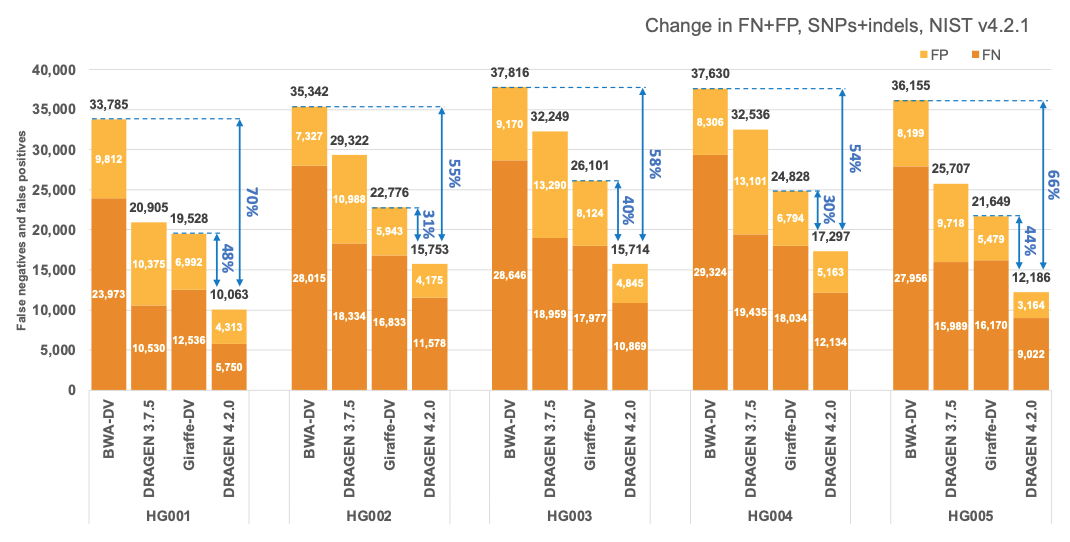
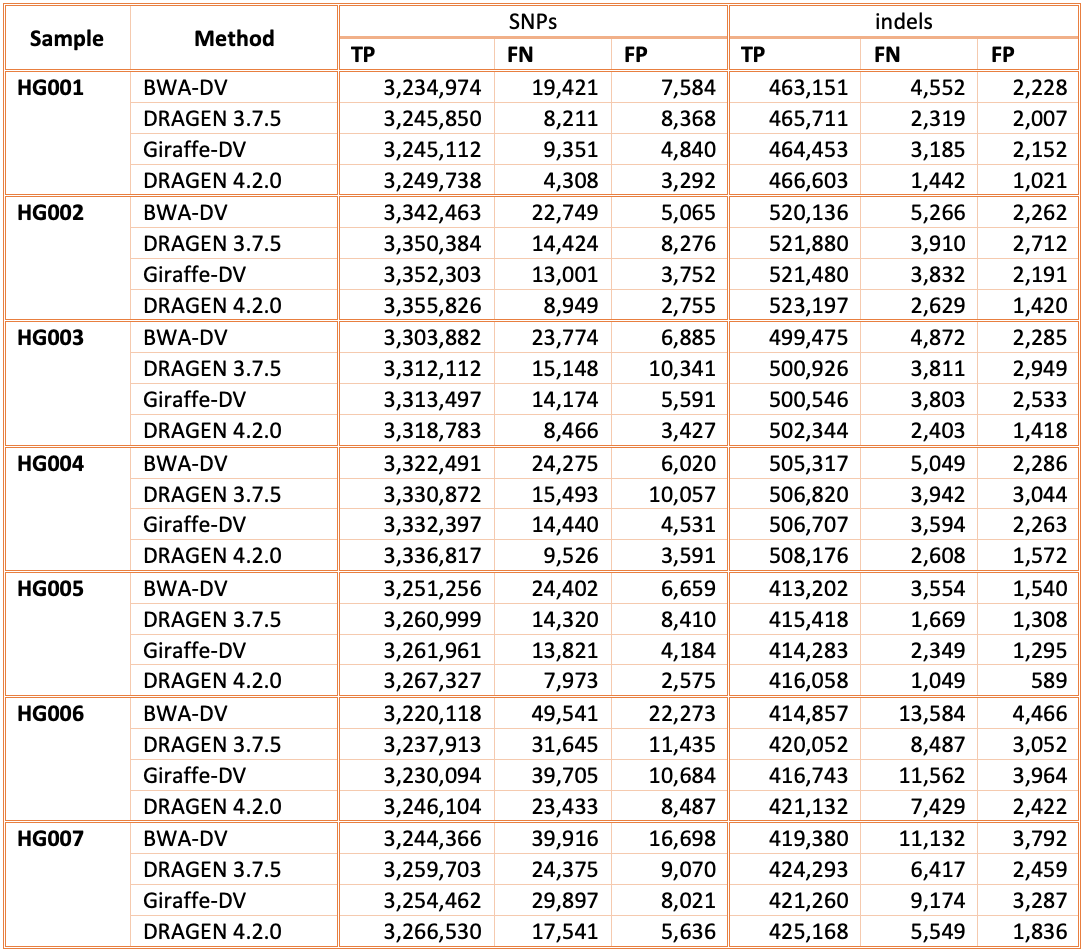
Accuracy on CMRG benchmarking regions
Figure 6 displays the accuracy of successive DRAGEN versions on the CMRG truth set,4 for SNPs and indels combined. The accuracy improvements observed in the NIST v4.2.1 All Benchmark Regions are also evident in the CMRG benchmarking set, resulting in an overall error reduction of 61%. This finding is consistent with the repetitive or polymorphic complexity of the genes in these CMRG regions. Specifically, introducing the multigenome reference in version 3.7.5 led to a 23% error reduction, followed by the v2 masking of the hg38 reference in version 3.10.4, which resulted in an additional 28% error reduction. The most recent reference and multigenome (graph) updates in version 4.2.0 contributed an additional 25% error reduction.
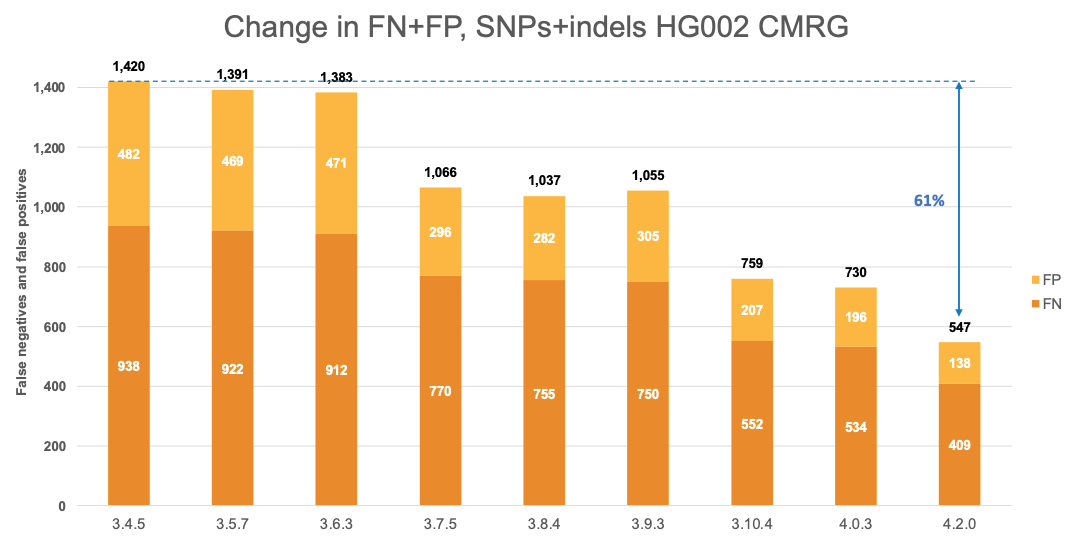
Comparison with the Human Pangenome Reference Consortium
In Figure 7 we extend the comparison of the performance of DRAGEN with the Human Pangenome Reference Consortium selected pipelines to the CMRG truth set. The results in the CMRG truth set follow the same trend as in the All Benchmark Regions, with DRAGEN v4.2.0 showing a combined error reduction of 50% compared to BWA-DV and 25% compared to Giraffe-DV. The error reduction breaks down to 55% and 25% on SNPs and 35% and 26% on indels for BWA-DV and Giraffe-DV, respectively.
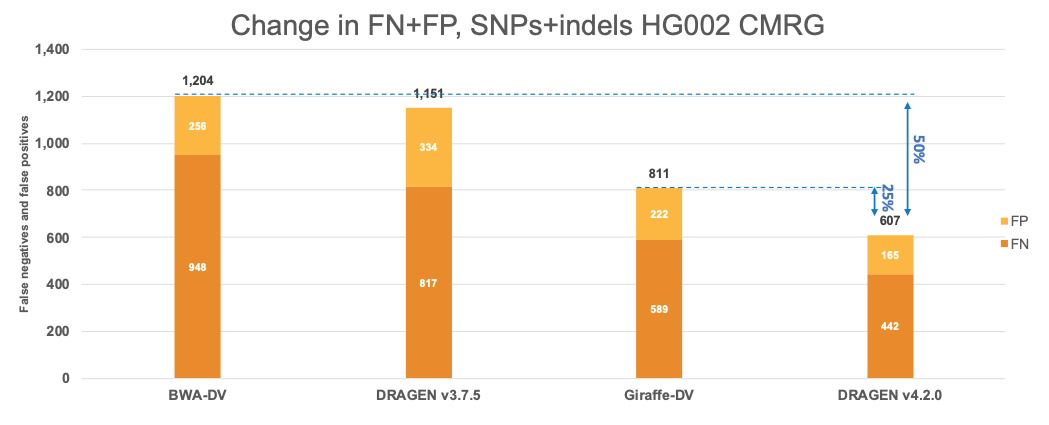
Evolution of key features across DRAGEN versions
Since 2020, DRAGEN’s germline variant calling accuracy has been substantially improved with the introduction of key features such as the multigenome (graph) reference (in 3.7.5), the machine learning recalibration (in 3.9.2 and made default in 4.0.3), and hg38 reference updates.14 As shown in Figure 8, these key features were refined and improved across DRAGEN versions. Please note that the definition of each hg38 reference label and DRAGEN multigenome (graph) version is given in Table 2, farther down.
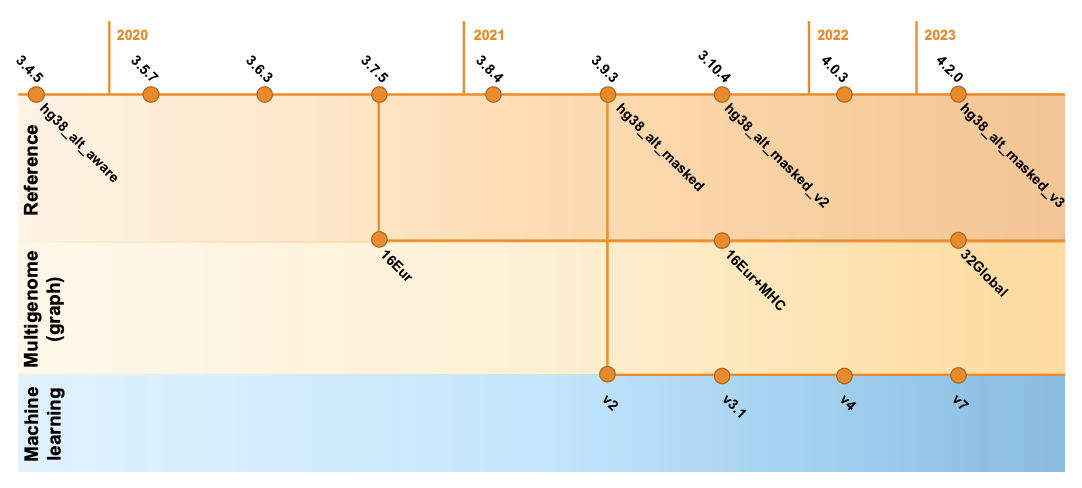
Multigenome (graph) reference
The multigenome (graph) reference in DRAGEN has been proven to drastically increase the mapping and small variant calling accuracy of Illumina reads in Difficult-to-Map Regions of the genome.15,16
In the DRAGEN multigenome (graph) reference, standard reference genomes hg38, hg19, and hg37d5 are augmented with carefully chosen population haplotype segments that help disambiguate the mapping in highly polymorphic and homologous regions through alternate paths known to commonly occur in the population. This essentially converts what was a flat, one-dimensional reference genome into a more complex, contoured map based on expectations laid out by the sequencing of many human genomes. For example, hg38 was augmented with 800,000+ contigs and 400,000+ SNPs in Difficult-to-Map Regions, covering more than 10% of the genome.
To capture more of the genetic diversity across the human population, the updated multigenome (graph) reference available in DRAGEN v4.2 replaces the original set of 16 population samples of European ancestry with 32 samples from different ancestries around the globe. With this new “v3” multigenome (graph) reference, more than 12% of the genome is boosted compared to the standard reference, a 20% improvement over the previous version with much less ethnicity bias.
Machine learning recalibration
Machine learning is another key component of the DRAGEN germline small variant accuracy.15 In DRAGEN v4.2, we extended the model training data to enhance the accuracy of variant calls across depth and assay configuration, including exome data. Additionally, we enhanced performance across reference genome options and introduced new features that improve indel calling and boost accuracy in noisy regions with dense pileups. Not satisfied with just improving the accuracy results, we were also able to optimize the performance of ML computing. The upgraded process is highly efficient, to the extent that enabling the ML option does not noticeably increase the runtime for whole-genome or exome datasets.
GRCh38/hg38 reference
The hg38 reference genome has undergone multiple revisions and improvements over time, as described in a previous post14 and in Genome Biology.17 The most recent reviews of the reference genome were driven by comparative analysis with the new complete human assembly18 of chm13 that identified false negative segmental duplications and unveiled previously unknown regions of the human genome. In the latest DRAGEN v.4.2, we recommend using “hg38-alt-masked-v3” and “hg38-alt-masked-v3-multigenome,” which incorporate the latest updates to the publicly available GRCh38 reference genomes.
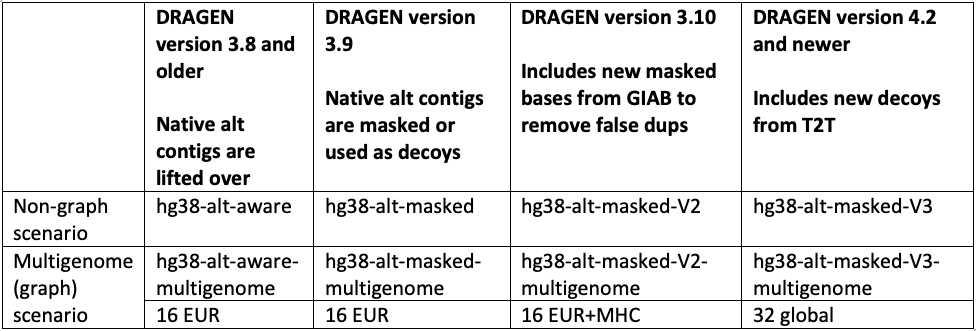
Note that the baseline assembly for all these references was downloaded from the European Bioinformatics Institute.19 We refer to ”16 EUR” as graph haplotypes from 16 individuals of European ancestry; ”32 global” as graph haplotypes from 32 individuals from Europe, Africa, Asia, and the Americas; and ”Native alt contigs” as 700+ "*_alt" and HLA contigs present in the GRCh38 reference genome.
In accordance with these public updates, we have incorporated additional sequences from chm13 and hs37d5 as decoy contigs in hg38-alt-masked-v3. These sequences were not included in GRCh38 due to collapsed duplication4,17 or unknown sequence gaps (N-masking of short arms of acrocentric chromosomes). By including these decoy contigs, we were able to enhance the accuracy of variant calling in several challenging genes (FANCD2, MAP2K3, KCNJ18, KMT2C), as well as regions of the Y chromosome.
Summary
DRAGEN v4.2 represents a culmination of multiple tireless efforts to shed light on the challenging regions of the genome. In this article, we have explored how improvements to the reference genome, multigenome (graph), and machine learning have enabled major leaps forward in SNP and indel calling accuracy across the regions of the genome that pose the greatest challenges. In previous posts we have explored how class-based callers like ExpansionHunter2 and gene-specific callers for genes like GBA13 are used to search for other types of challenging variations.
DRAGEN v4.2 consistently demonstrates impressive accuracy in calling germline small variants, surpassing itself over previous versions, according to testing conducted on both the NIST v4.2.1 All Benchmark Regions and the CMRG regions. The platform is notable for its accuracy, speed, scalability, ubiquity, and comprehensiveness, making it an essential tool for all genomics analyses. With its unique features, DRAGEN is a potent resource for researchers and scientists seeking to conduct comprehensive and efficient analyses of genomic data.
The results presented in this post emphasize the crucial point that the use of Illumina's high-quality sequencing technology in conjunction with DRAGEN's informatics leads to variant calling of exceptional accuracy, including in challenging regions.
DRAGEN v4.2 is set to release in June 2023.
For more information or a DRAGEN trial license for academic use, please contact dragen-info@illumina.com.
DRAGEN command lines
DRAGEN resource files for previous versions of DRAGEN can be downloaded here. We report the command line options we used in our tests in Table 3 and Table 4. Reference hash tables and ML models are reported in Table 5.
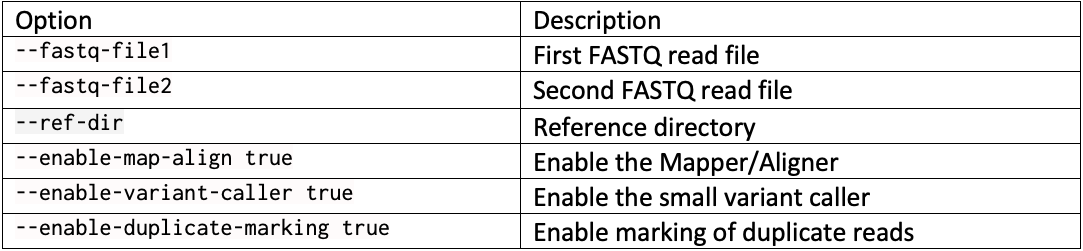

Version |
Reference hash table link |
ML model link |
3.4.5 |
not applicable |
|
3.5.7 |
not applicable |
|
3.6.3 |
not applicable |
|
3.7.5 |
not applicable |
|
3.8.4 |
not applicable |
|
3.9.3 |
||
3.10.4 |
||
4.0.3 |
V4.0 (default) |
|
4.2.0 |
hg38_alt_masked_multigenome_v3 |
V7.0 (default) |
Table 5. Links to the reference hash table and ML model for each applicable version.
Complete command line example with … replacing run-specific parameters such as the reference directory, the output directory, and the sample FASTQs: dragen --fastq-file1 … --fastq-file2 … --RGSM HG002 –RGID HG002 --ref-dir … --output-file-prefix HG002 --events-log-file dragen_events.csv --output-directory … --generate-sa-tags true --enable-vcf-compression true --enable-variant-caller true --enable-map-align true --enable-map-align-output true --enable-sort true --enable-duplicate-marking true --enable-bam-indexing true
Concordance with NIST truth is performed using the RTG toolkit as described in Nature Biotechnology.20 A comparison example command is as follows: java -Djava.awt.headless=true -Dtalkback=false -Dusage=false -Xmx40g -jar RTG-3.9.1.jar vcfeval -b <truth>.vcf.gz -c <query>.vcf.gz -t <tmp_dir> -o <output_dir> --output-mode annotate --vcf-score-field QUAL --bed-regions <truth>.bed -Z --sample <truth sample>,<query sample> --ref-overlap
For all DRAGEN runs, we use the recommended <sample>.hard-filtered.vcf.gz VCF output file which results in best f1-score measure.
References
- N. D. Olson et al., “PrecisionFDA Truth Challenge V2: Calling variants from short and long reads in difficult-to-map regions,” Cell Genomics, vol. 2, no. 5, p. 100129, 2022, doi: https://doi.org/10.1016/j.xgen.2022.100129.
- S. Strom, C.-L. Mead, D. Letchworth, V. Onuchic, and M. Bekritsky, “Fully featured genome: expanding the hunt for genomic variation with DRAGEN STR.” https://www.illumina.com/science/genomics-research/articles/str-expansionhunter.html
- S. Strom, “Using DRAGEN for Gaucher and Parkinson disease research: resolving GBA1 variants using PCR-free whole-genome sequencing.” https://www.illumina.com/science/genomics-research/articles/using-dragen-for-gaucher-and-parkinson-disease-research--resolvi.html
- J. Wagner et al., “Curated variation benchmarks for challenging medically relevant autosomal genes,” Nat Biotechnol, vol. 40, no. 5, pp. 672–680, 2022, doi: 10.1038/s41587-021-01158-1.
- J. Wagner et al., “Benchmarking challenging small variants with linked and long reads,” Cell Genomics, vol. 2, no. 5, p. 100128, May 2022, doi: 10.1016/J.XGEN.2022.100128.
- H. Li, “Aligning sequence reads, clone sequences and assembly contigs with BWA-MEM,” Mar. 2013, Accessed: May 02, 2023. [Online]. Available: https://arxiv.org/abs/1303.3997v2
- G. A. Van der Auwera and B. D. O’Connor, Genomics in the Cloud : Using Docker, GATK, and WDL in Terra. O’Reilly, 2020.
- R. Poplin et al., “A universal SNP and small-indel variant caller using deep neural networks,” Nature Biotechnology 2018 36:10, vol. 36, no. 10, pp. 983–987, Sep. 2018, doi: 10.1038/nbt.4235.
- “PDR: precisionFDA Truth Challenge V2: Calling variants from short- and long-reads in difficult-to-map regions.” https://data.nist.gov/od/id/mds2-2336 (accessed Apr. 27, 2023).
- S. Zhao, O. Agafonov, A. Azab, T. Stokowy, and E. Hovig, “Accuracy and efficiency of germline variant calling pipelines for human genome data,” Scientific Reports 2020 10:1, vol. 10, no. 1, pp. 1–12, Nov. 2020, doi: 10.1038/s41598-020-77218-4.
- W.-W. Liao et al., “A draft human pangenome reference,” Nature 2023 617:7960, vol. 617, no. 7960, pp. 312–324, May 2023, doi: 10.1038/s41586-023-05896-x.
- J. Sirén et al., “Pangenomics enables genotyping of known structural variants in 5202 diverse genomes,” Science, vol. 374, no. 6574, pp. 1-11, Dec. 2021, doi: 10.1126/SCIENCE.ABG8871.
- “A draft human pangenome reference – Variant calls” s3://human-pangenomics/publications/PANGENOME_2022/DeepVariant/samples (accessed May 18, 2023).
- S. Catreux et al., “Demystifying the versions of GRCh38/hg38 Reference Genomes, how they are used in DRAGEN and their impact on accuracy.” https://www.illumina.com/science/genomics-research/articles/dragen-demystifying-reference-genomes.html
- S. Catreux et al., “DRAGEN Sets New Standard for Data Accuracy in PrecisionFDA Benchmark Data.” https://www.illumina.com/science/genomics-research/articles/dragen-shines-again-precisionfda-truth-challenge-v2.html
- R. Mehio et al., “DRAGEN Wins at PrecisionFDA Truth Challenge V2 Showcase Accuracy Gains From Alt-aware Mapping and Graph Reference Genomes.” https://www.illumina.com/science/genomics-research/articles/dragen-wins-precisionfda-challenge-accuracy-gains.html
- S. Behera et al., “FixItFelix: improving genomic analysis by fixing reference errors,” Genome Biol, vol. 24, no. 1, p. 31, 2023, doi: 10.1186/s13059-023-02863-7.
- S. Nurk et al., “The complete sequence of a human genome,” Science (1979), vol. 376, no. 6588, pp. 44–53, Apr. 2022, doi: 10.1126/SCIENCE.ABJ6987.
- “GRCh38 Full Analysis Set Plus Decoys HLA.” http://ftp.1000genomes.ebi.ac.uk/vol1/ftp/technical/reference/GRCh38_reference_genome/GRCh38_full_analysis_set_plus_decoy_hla.fa (accessed Mar. 30, 2023).
- P. Krusche et al., “Best practices for benchmarking germline small-variant calls in human genomes,” Nature Biotechnology 2019 37:5, vol. 37, no. 5, pp. 555–560, Mar. 2019, doi: 10.1038/s41587-019-0054-x.